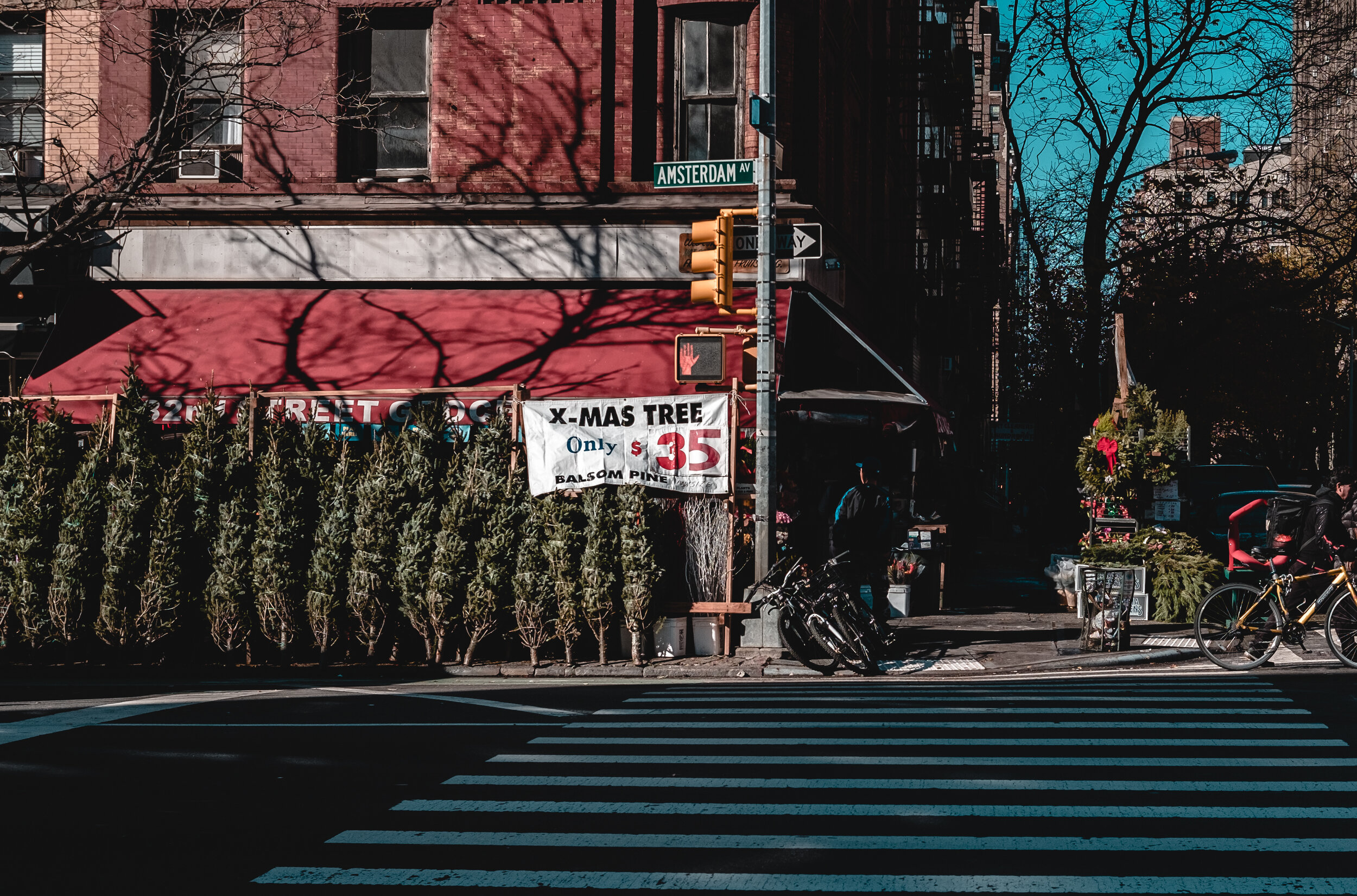
Ecommerce & Logistics Case Study
Project Background
In 2015, I cofounded DC Tree Delivery— a local Christmas tree delivery/removal service. After 2 profitable seasons with high ratings from customers, we hit major growing pains as we tried to increase sales volume in Season 3 (Oct 2017 - Jan 2018). Ultimately we ended up netting our first season “in the red”. For Season 4, the goal was to increase the average profit margin of our profitable seasons by at least 5% without increasing sales volume or drastically raising prices.
“I have nothing but words of praise for this fantastic company. Beautiful tree; timely delivery and removal; efficient and friendly team. I give them a 10-plus. See you next Christmas season, and thank you for everything!”
— Yelp Reviewer (5 stars)
Project Summary
Goal
The goal was to increase the average profit margin of our profitable seasons by at least 5% without increasing sales volume or drastically raising prices.
To identify the areas of greatest impact, I used Excel to analyze 3 years of historical data from our bank, delivery routing software, Shopify, and Google analytics data. I then built an Excel model to manipulate input variables in order to test certain scenarios. This exercise revealed the most effective levers I could pull to achieve our goal.
Approach
From our historical data I discovered that 70% of our expenses came from transportation (truck rentals) and hourly labor. To reduce our transportation and labor costs, we needed to prioritize the following:
Reduce miles driven
Reduce the number of days we held rentals
Reduce total hours worked (as opposed to reducing wages)
Increase deliveries/hour to bring greater efficiency to the overall operation
Priorities
Solutions
After brainstorming and model testing ways to increase profits while reducing sales volume, these 5 potential solutions surfaced (see appendix for more details):
Restrict delivery to just the top 10 zip codes, to increase density & reduce average workday hours and overall time on the road.
Only sell our top 3/10 products, ordering initial stock quantities based on each’s profitability to further boost profit/delivery.
Reduce idle time (time spent on the clock not delivering trees) by boosting capacity early in the season with extra crews then scaling down quickly as demand dipped. This would ensure every hour we paid an employee was being used effectively.
Raise prices on our loss leader— tree removals to increase revenue without customers feeling like they were losing value.
Move our base of operations closer to our delivery hotspots to further save on transportation costs.
Outcome
In the end, the steps we took led to our best season yet. Our profit margin increased 9%, work days were 1-2 hours shorter on average, and we had a much shorter selling season after selling out early.
Even more rewarding, we were able to achieve these results while increasing staff wages and making a donation to a local nonprofit helping the homeless stay warm.
Appendix
(Data & Photo Gallery)
From financial data, 70% of expenses came from transportation and labor.
Transportation: The majority of transportation costs came from truck rentals comprising of a base daily rental cost and a per mile cost. To reduce costs, we needed to reduce miles driven and reduce the number of days we held rentals.
Labor: To reduce hourly labor costs we could lower wages, reduce total hours worked, or increase the amount of work done/hour (# of deliveries/hour). We did not want to reduce wages so we needed to reduce total work hours and increase deliveries/hour to compensate.
Ecommerce & Logistics Data
From Shopify and WorkWave (routing software) I found data that confirmed my hypotheses and provided insights on opportunities for optimization.
50% of orders came from just 7.4% of service area zip codes
75% of revenue came from just 30% of the products sold
Idle hours peaked when sales volume dipped